Protein modeling in the AlphaFold era
1 The history of protein structure modeling is told by a competition (CASP)
Every two years since 1994, groups in the field of structural bioinformatics have conducted a worldwide experiment in which they predict a set of unknown protein structures in a controlled, blind test-like competition and compare their results with the experimentally obtained structures. That is the CASP or Critical assessment of Protein Structure Prediction.
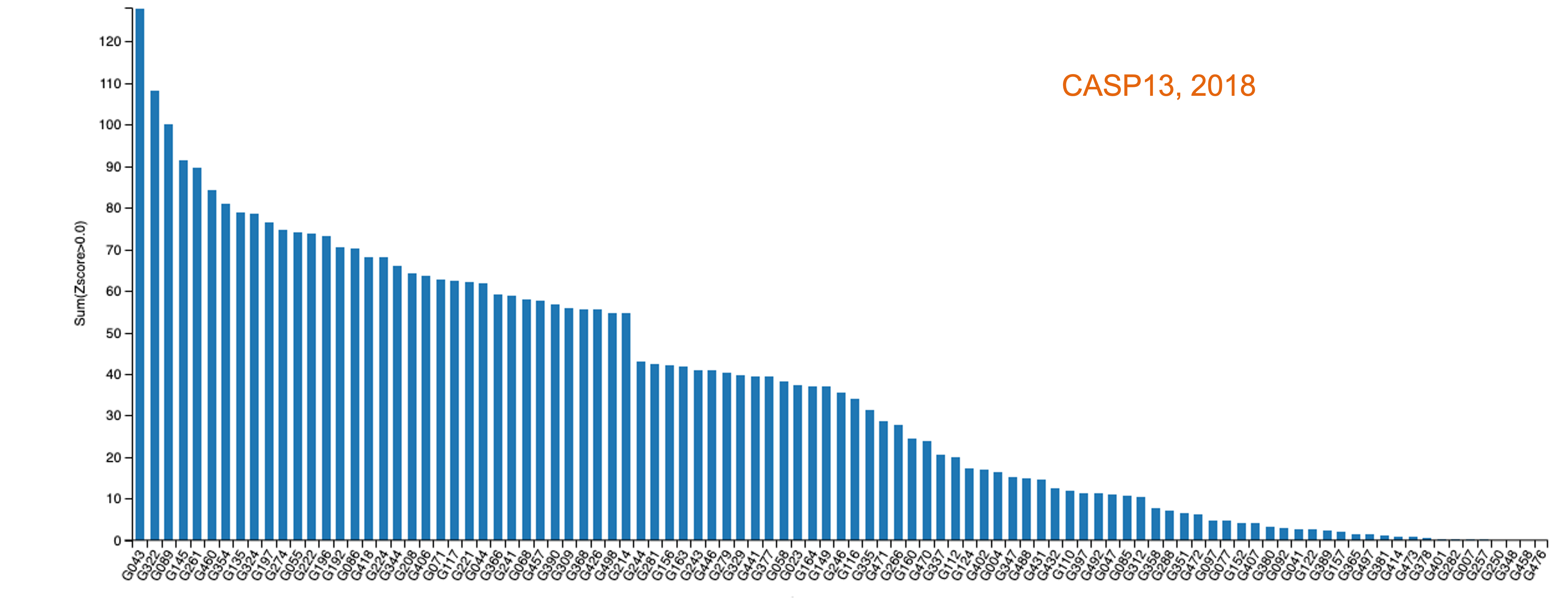
The best research groups in the field test their new methods and protocols in the CASP. However, at CASP13 (2018), an AI company called Deepmind (Google Subsidiary) entered the scene. Their method, named Alphafold (Senior et al. 2020) clearly won CASP13. Alphafold (v.1) implemented improvements in some recently used approaches and created an entirely new pipeline. Instead of creating contact maps from the alignment and then folding the structure, they used an MRF unit (Markov Random Field) to extract the main features of the sequence and MSA in advance and process all this information into a multilayer NN (called ResNet) that also predicted distance probabilities instead of contacts, resulting in high accuracy. Then, Alphafold uses all the possibly obtained information to create the structure and then improve it by energy minimization (steepest descent method) and substitution of portions with a selected DB of protein fragments.
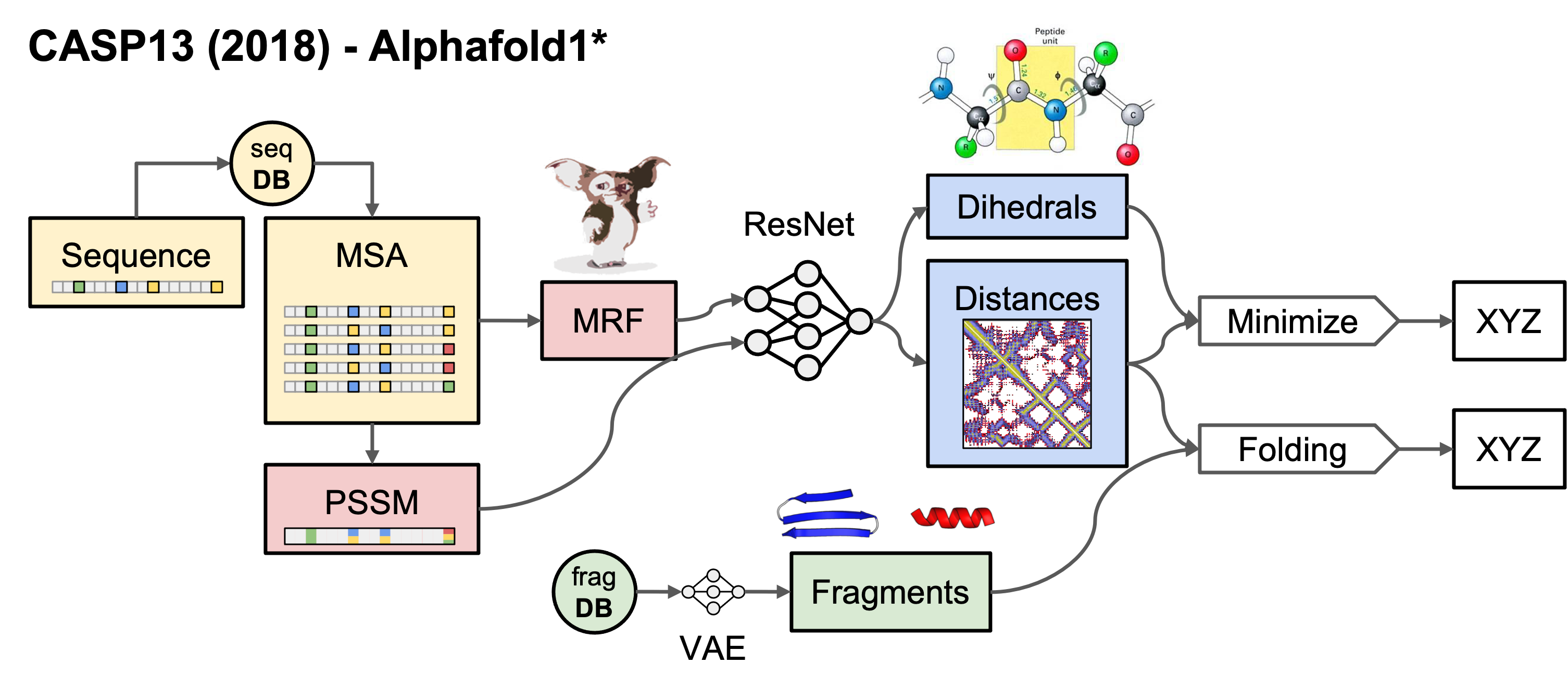
After Alphafold, similar methods were also developed and made available to the general public, like the trRosetta (Yang et al. 2020), from the Baker lab, available in Rosetta open source software and in the Robetta server. This led to some controversy (mostly on Twitter) about the open access to the CASP software and later on DeepMind publishes all the code on GitHub.
2 CASP14 or when protein structure prediction come to age for (non structural) biologists
There was a lot of hype in CASP14 and the guys from DeepMind did not disappoint anyone. Alphafold2 left all competitors far behind, both in relative terms (score against the other groups) and in absolute terms (lowest alpha-carbon RMSD). As highlighted earlier, the accuracy of many of the predicted structures was within the margin of error of the experimental determination methods (see for instance Mirdita et al. 2022).
Deepming took some time (eight months, which is an eternity nowadays) to publish the method (Jumper et al. (2021)) and to release the code on Github, but other new methods, such as RoseTTAfold (Baek et al. (2021)) and C-I-Tasser (Zheng et al. (2021)) were able to obtain similar results and were available on public servers, which may have pushed Deepmind to make it all available to the scientific community1. Not surprisingly, a group of independent scientists (Sergey Ovchinnikov, Milot Mirdita, and Martin Steinegger), decided to implement Alphafold2 in a Colab notebook, called ColabFold Mirdita et al. (2022), which freely available online using Google Colab notebooks platform. Other free implementations of Alphafold have been and are available, but ColabFold has been the most widely discussed and well known. They implemented some tricks to speed up the modeling, most notably the use of MMSeqs2 (developed by Martin Steinegger’s group) to search for homologous structures on Uniref30, which made Colabfold a fast method that made all the previous advanced methods almost useless. This was the real breakthrough in the field of protein structure prediction, making Alphafold accessible and, also very importantly, facilitated the further development of the method, implementing very quickly new features, like the prediction of protein complexes, which was actually first mentioned on Twitter and then led to several new dedicated methods, including AlphaFold-multimer (Evans et al. 2022) from Deepmind or AlphaPullDown (Yu et al. 2023).
3 Alphafold as the paradigm of a New Era
Why is Alphafold so freaking accurate?
The philosophy behind Alphafold v.2 (from now on, just Alphafold) and related methods is to treat the protein folding problem as a machine learning problem, similar to image processing. In all of these problems, the input to the Deep Learning model is a volume (3D tensor). In the case of computer vision, 2D images expand as volumes because of the RGB or HSV channels. Similarly, in the case of distance prediction, predicted 1D and 2D features are transformed and packed into a 3D volume with many channels of inter-residue information (Pakhrin et al. 2021).
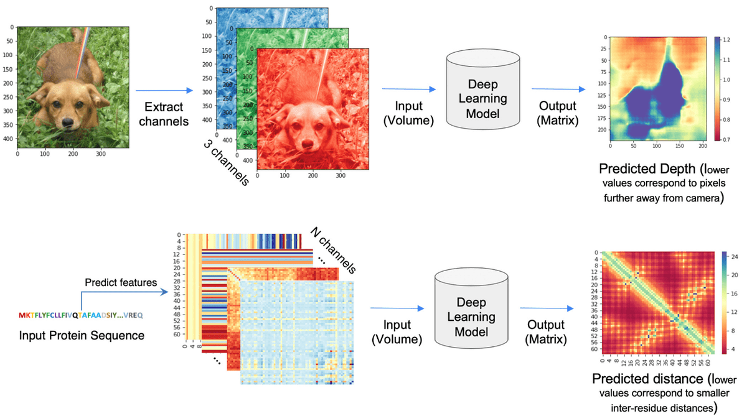
Alphafold can be explained as a pipeline with three interconected tasks (see picture below). First, in contrast to Alphafold v.1, the input to Alphafold is a “raw” MSA, i.e., the deep learning network extracts the co-evolutionary information directly from the MSA. It queries several databases of protein sequences and constructs an MSA that is used to select templates. This can be a limiting step, affecting the speed of modeling (see below), but it can be also related to model accuracy, as has been recently shown in CASP15 (Lee et al., n.d.; Peng et al. 2023).
In the second part of the diagram, AlphaFold takes the multiple sequence alignment and the templates, and processes them in a transformer that they named Evoformer. This process has been referred by some authors as inter-residue interaction map-threading (Bhattacharya et al. 2021). The objective of this part is to extract layers of information to generate residue interaction maps. The Evoformer refines both its understanding of the MSA and the predicted 3D structure in an iterative process. Improved predictions of amino acid interactions help refine the MSA representation, and vice-versa.
The AF2 transformer architecture allows the model to process all amino acids in a protein sequence simultaneously, rather than one at a time, through a technique known as the attention mechanism. This is a significant advantage because it enables the model to capture long-range relationships between amino acids. Essentially, the attention mechanism helps a neural network to concentrate on the most relevant parts of its input when making predictions. For proteins, this means the network can learn which amino acids are likely to interact with each other, even if they are far apart in the sequence. It’s akin to reading a lengthy document; you don’t focus equally on every word but rather on the key phrases that are crucial for understanding the overall meaning.
Importantly, in the AF2 Evoformer, this process is iterative and the information goes back and forth throughout the network. At each recycling step, the model refines its predictions of amino acid relationships, leading to a more accurate structure (the original model uses 3 cycles). As explained in the great post from Carlos Outerial at the OPIG site:
This is easier to understand as an example. Suppose that you look at the multiple sequence alignment and notice a correlation between a pair of amino acids. Let’s call them A and B. You hypothesize that A and B are close, and translate this assumption into your model of the structure. Subsequently, you examine said model and observe that, since A and B are close, there is a good chance that C and D should be close. This leads to another hypothesis, based on the structure, which can be confirmed by searching for correlations between C and D in the MSA. By repeating this several times, you can build a pretty good understanding of the structure.
The third part of the pipeline is the structure building module, which uses the information from the previous steps to construct a 3D model structure protein of the query sequence. This network will give you a single model, without heavy energy optimization steps (only a relax protocol). Model building is based in a new concept of 3D structures generation, named IPA (Invariant Point Attention) and the use of a curated list of parametrized list of torsion angles to generate the side chains. IPA allows the network to maintain rotational and translational invariance, which is crucial for accurate 3D structure prediction. Earlier attempts to develop and end-to-end method were unsuccessful because the structure representation was not optimal. Even methods implemented after AlphaFold, like RoseTTAFold, use less efficient methods and often predict very quickly and accurately the backbone coordinates but require external programs to generate an all-atoms model.
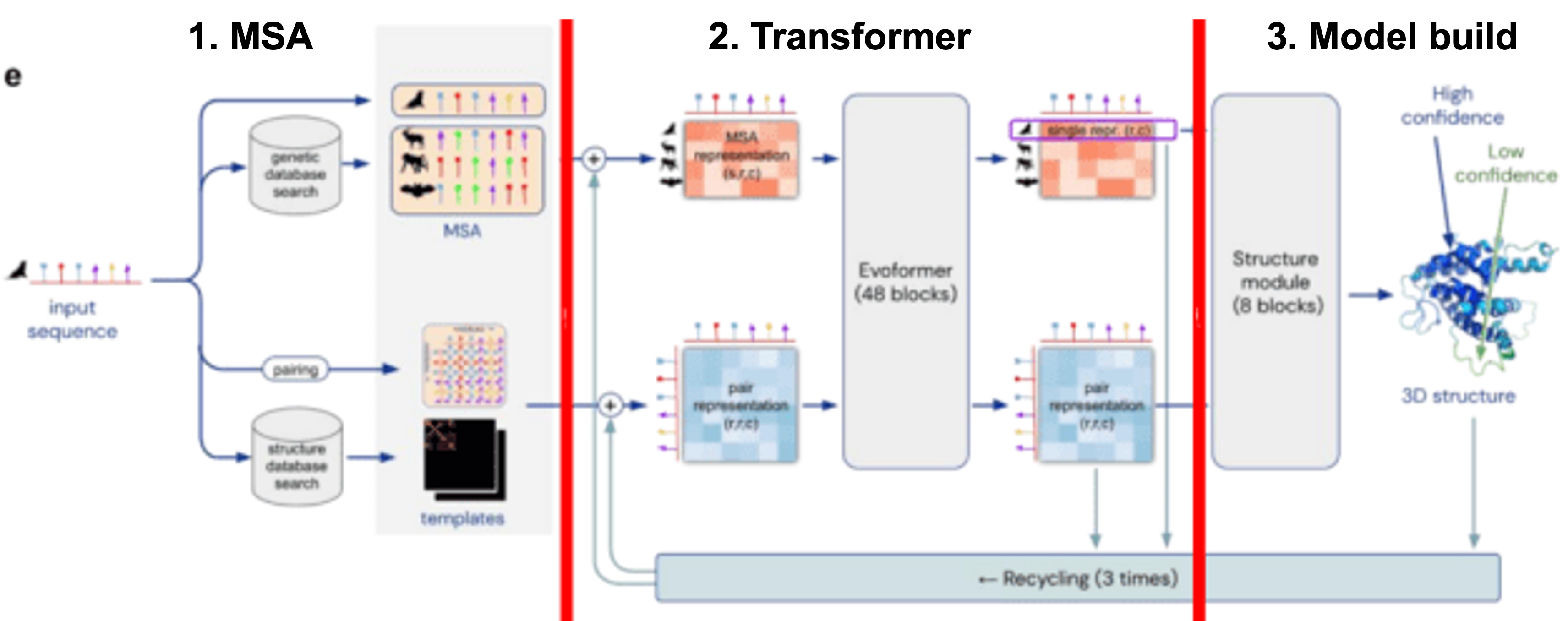
Like for most of the previous methods Alphafold would give your better results with proteins with related structures known and with a lot of homologs in Uniref databases. However, comparing to nothing, it will likely give you (limited) useful results for the so-called “dark genome”. I work with phages and bacterial mobile elements, and sequencing that is often frustrating as more than 50% of the proteins have no homologous in the database. So you have a bunch of proteins of unknown function… However, as we do know that structure is more conserved than sequence, we may use the structure to find out the function of our dark proteins. There are a few resources for this, I’d suggest you to try FoldSeek (Kempen et al., n.d.) and Dali (Holm 2022) servers. You can upload the PDB file of your model and search for related structures in RCSB PDB database and also in Alphafold database.
FoldSeek needs only a few seconds/minutes and is therefore faster than Dali. Therefore, it is better to use Dali for some selected searches that require a double check or a more reliable result, even if it may take a few days.
As mentioned above, Colabfold aims to make the process faster by using MMSeqs in the first block. Additionally, the number of recycling steps can also be adapted. Moreover, different Colabfold notebooks have been developed (and evolved) to allow some customization and other feature, like batch processing of multiple proteins avoiding recompilation and identification of protein-protein interactions (Mirdita et al. 2022).
Alphafold models can be evaluated by the mean pLDDT, a per-residue confidence metric. It is stored in the B-factor fields of the mmCIF and PDB files available for download (although unlike a B-factor, higher pLDDT is better). The model confidence can vary greatly along a chain so it is important to consult the confidence when interpreting structural features. Very often, the lower confidence fragments are not product of a poor prediction but an indicator of protein disorder (Wilson, Choy, and Karttunen 2022). Additionally, AF2 calculates the predicted template modeling, or TM-scores (pTM) based on a pairwise error prediction, and is used to calculate the predicted aligned error (PAE) that estimates the error of the position of each amino acid. Interestingly, the PAE can be used as a predictor for protein dynamics, as PAE correlates with distance variations from the MD simulations (Guo et al. 2022).
Alphafold also partnered with EMBL-EBI and Uniprot and generated a huge curated database of proteins from model organisms (Varadi et al. 2022), the Alphafold database. This is an amazing resource that may be also very helpful for you. Just consider that this database increased from 48% to 76% the fraction of human proteome with structural data, and also it also means great increases in the case of other model organisms, like, including microorganisms and plants (Porta-Pardo et al. 2022).
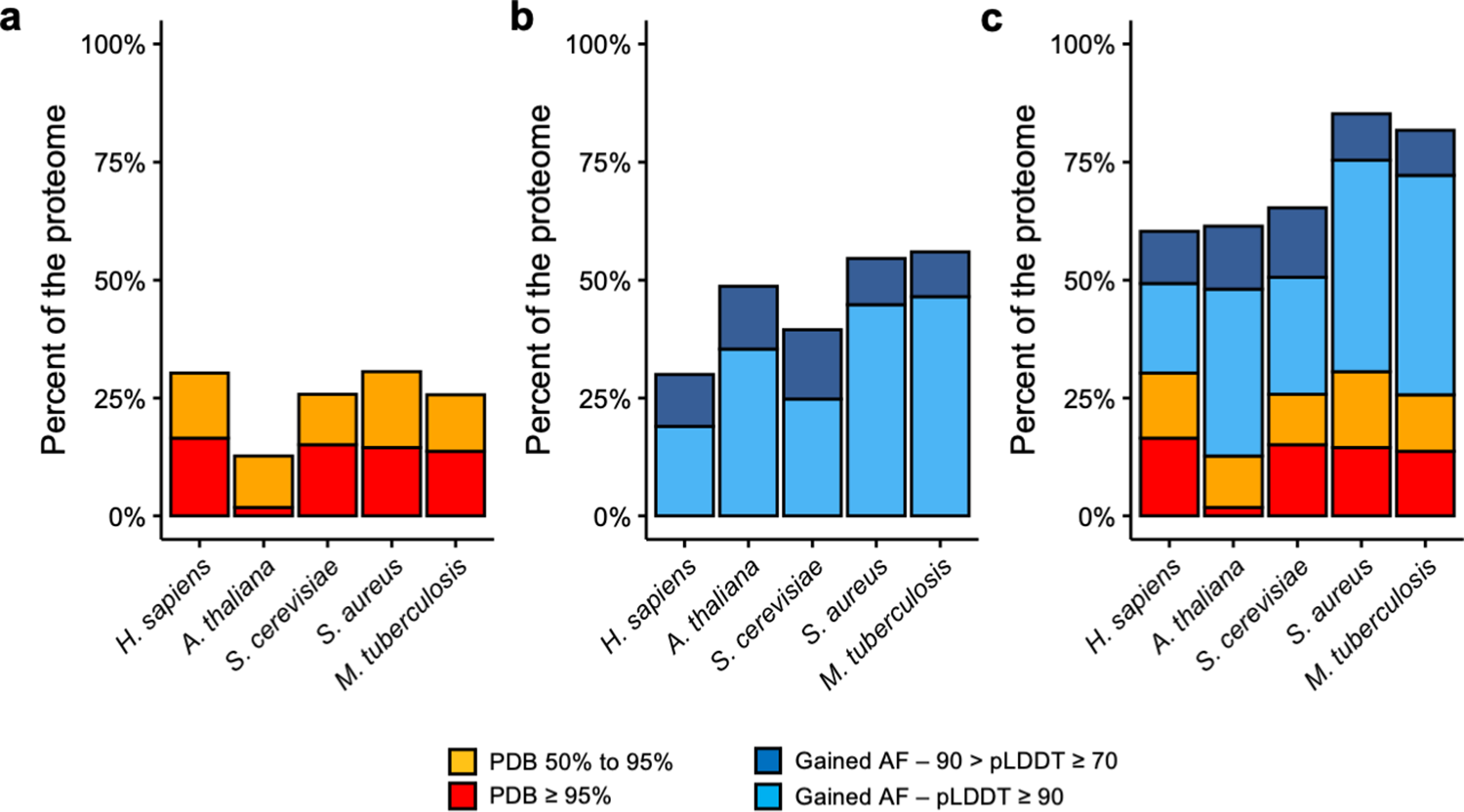
Wanna try Alphafold?
Section under construction!
As mentioned above, the grand breakthrough of Alphafold would not have been the same without the Colabfold, a free open tool that made the state-of-the-art of AI-fueled protein prediction available to everyone.
The Colabfold repository on GitHub contains links to several Python notebooks developed on Google Colab, a platform to develop and share Python scripts on a Jupyter Notebook format. Notebooks are very important also for reproducibility in computer sciences, as they allow you to have the background and details and the actual code in a single document and also execute it. You can share those notebooks very easily and also update quickly as they are stored in your Google Drive.
Colabfold allow you to run notebooks of Alphafold, RoseTTAfold, and ESMFold for specific applications, allowing even to run a bunch of proteins in batch. You can see a more detailed description in Mirdita et al. (2022). We are using the Alphafold2_mmseqs2 notebook, that allow you most of the common features. You need to allow Colab to use your Google account.

Then paste your sequence and chose a name. For more accurate models you can click “use_amber” option. It will run a short Molecular Dynamics protocol that ultimately optimize the modeling, but it will also take some more time, so better try at home.
As you can see, an this is a recent feature, you can also add your own template, which combines the classical “homology modeling” based upon a single template with the advanced AlphaFold model construction method. That will safe time, but of course without any guarantee. If you have a template of a related protein, like an alternative splicing or a disease mutant, I’d advise you to try with and without the template. You may surprise.
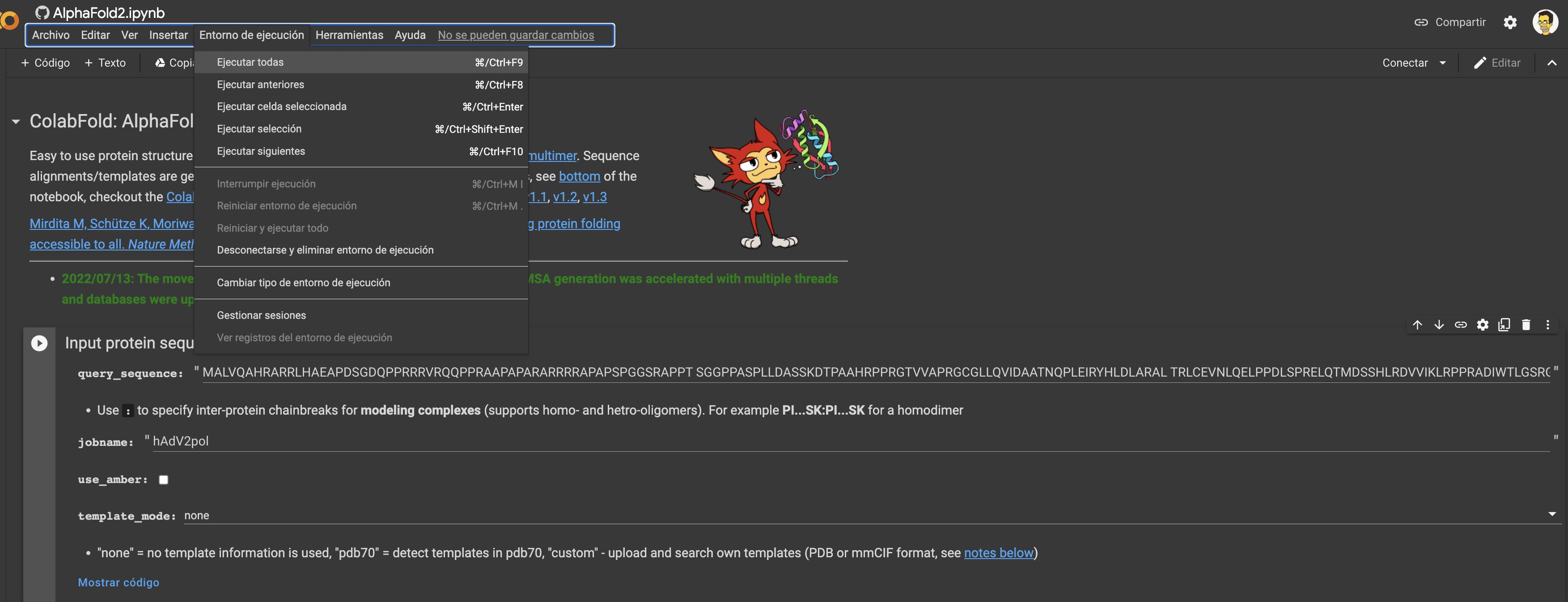
At this point, you may execute the whole pipeline or may some more customization. MSA stage can be also optimized to reduce execution time, by reducing database or even by providing your own MSA. Very often you may want to fold a protein with different parameters, which may very convenient to reuse an MSA from a previous run (although they recently updated servers for MMSeqs and made it really faster). If your proteins are in the same operon or by any other reason you think that they should have co-evolved, you prefer a “paired” alignment. But you can always do both.
Advanced settings are specially needed for protein-protein complexes. Also the number of recycling steps will improve your model, particularly for targets with no MSA info from used databases. Then you can just get your model (and companion info and plots) in your GDrive or download it.
What do you think is the ideal protein for AlphaFold? Do you think homology modeling is dead?
Now what? The Post-AlphaFold era (2022-2023)
As mentioned earlier, RoseTTAFold was released at the same time as AlphaFold’s paper and code, although it is clearly inspired by AlphaFold’s capabilities in CASP14. It is based on a three-track network, and recent implementations have allowed prediction of protein interactions with nucleic acids. Other methods such as AlphaFold and RoseTTAFold were released later, as were OpenFold and UniFold, which are based on the PyTorch Transformers AI framework. More recently, RoseTTAFold2, which extends the three-track architecture over the whole network and incorporate other new advances and AlphaFold tricks, like the FAPE (frame aligned point error) or recycling steps during the training, giving rise to an end-to-end model equivalent in accuracy to AF2 for monomers and AF2-multimer for complexes, with better computational scaling on proteins and complexes larger than 1000 residues (Baek et al., n.d.).
The use of MSA has been cited as a limitation for AlphaFold and related methods. However, the predictions are significantly worse without MSA or with MSAs without depth. One way to improve predictions is to use a protein natural language model (PLMs), i.e., a model trained to predict sequence from sequence and not dependent on good MSAs. Omegafold and ESMfold (also in Colabfold, here and here, respectively) are two new implementations that require only a single sequence. While AlphaFold2 uses deep learning with multiple sequence alignments (MSAs) to infer protein structures, PLMs learn the “language” of protein sequences directly, representing amino acid sequences. Each amino acid (e.g., alanine, glycine) is represented as a “token,” a numerical representation that the model can process. By training on extensive datasets of protein sequences, PLMs learn the patterns and relationships between amino acids, enabling them to predict 3D structures from single sequences. Essentially, PLMs treat amino acid sequences as “sentences,” where each amino acid is a “word”. This method offers advantages in speed and applicability, particularly for proteins without sufficient sequence homology. However, they may have lower overall accuracy compared to AlphaFold2 when MSAs are available. PLMs perform efficiently when using a single sequence, often surpassing AlphaFold in this scenario. Nevertheless, although they can outperform AF2, since PLMs are trained on existing sequences, these methods still show reduced performance on orphan sequences. That is, the language models seem to replicate the training MSAs (Elofsson 2023).
The appearance of ESMfold and the companion Metagenomic Atlas (Lin et al. 2023) was seen as a new step that could trigger a new revolution in the field, since it was developed by scientists at META (Callaway 2022). So it was now a sort of Google versus Facebook battle for the most powerful AI methods for protein modeling. Last summer, however, we learned that META had decided to discontinue the ESM project.
AlphaFold was again the protagonist of CASP15, which took place in 2020, but it did not overwhelm the rest like in 2018.
AlphaFold was used in some form in more than half of the protocols, and while the standard AlphaFold method performed better than many other methods, several groups achieved significant improvements for monomeric proteins and protein assemblies. In short (see Elofsson (2023)), we learned at CASP15 that there are two main ways to improve AlphaFold: (1) more efficient use of templates, increasing the size of the database or sampling through more efficient MSAs, or (2) hacking AlphaFold to use dropouts to generate thousands of models for each target, which increases computational time but also increases the chances of better models. Other small improvements have been proposed, such as refinement steps (Adiyaman et al. 2023) or using improved template search or new scoring capabilities based on Voronoi surfaces or Deep Learning.
2021-2023 - Short summary in one wire
Corollary 1: Did Deepmind “fold” Levinthal’s paradox?
The development of Alphafold and the Alphafold structures Database in collaboration with EMBL-EBI was been the origin of a New Era. Moreover, in a further turn, the Metagenomic Atlas by Meta AI uncovers virtually the whole protein space. Thanks to these milestones the coverage of the protein structure space has been greatly increased (Porta-Pardo et al. 2022), which virtually close the sequence-structure gap. Since 2020, many scientific publications and journals worldwide published long articles about the meaning of this breakthrough in science and its applications in biotechnology and biomedicine2 and DeepMind claimed to have Solved a 50-years Grand Challenge in biochemistry.
In other words, after AlphaFold, would it no longer be necessary to perform X-ray crystallography or nuclear magnetic resonance? First, AlphaFold models can be used in electron density maps and help solve complex cases. Thus, the new framework helps crystallographers focus their work on the most unresolved and difficult structures, such as coiled-coils or holoproteins, which cause modulations and challenges in the development of crystallographic methods.
However, some scientists argued that Alphafold2 and RoseTTAfold actually cheat as they do not really solve the problem but generate a deep learning pipeline that is able to bypass the problem (Pederson 2021). In agreement with that, it has been shown that machine learning methods actually do not reproduce the expected folding pathways while improving the structures during the recycling steps (Outeiral, Nissley, and Deane, n.d.).
In conclusion, I believe that the Levinthal paradox is not (yet) fully solved, although it seems to be close (Al-Janabi 2022). Practically, it is solved for most of the protein space, but if your protein does not have a homolog in the databases, you will still have some open questions.
4 Protein hallucination and diffusion models
Biochemists and Chemists have dreamed of designing proteins with customizable properties for decades. First successful protein designs were published in the late 1990s and several breakthrough by Baker’s lab pushed forward the idea. More recently, artificial intelligence is starting a revolution in this field, and it will radically change it forever (reviewed in Notin et al. (2024)).
Soon after AF2 and RoseTTAFold publication, they were used to “hallucinate” new proteins. In December 2021, Baker and his colleagues reported expressing 129 of these hallucinated proteins in bacteria, and found that about one-fifth of them folded into something resembling their predicted shape. Then, in 2023, they introduced RFdiffussion, a new paradigm in protein design, based in AI diffusion models. Imagine starting with a cloud of randomly positioned atoms (pure noise). During training, diffusion models apply usually-random noise to a distribution of data until it resembles pure static, for example. The model then learns to reverse this process, generating final data points representative of the original data distribution. This process is guided by learned patterns of how atoms and molecules interact, allowing the model to “denoise” the initial random arrangement into a realistic biomolecular complex. The model learns to reverse a “diffusion process,” where noise is progressively added to known biomolecular structures. By learning to undo this process, the model can generate new structures from scratch.
More recently several groups have combined diffusion models with NLPs to develop improved methods to design new proteins. Protein sequences can be described as a concatenation of letters from a chemically defined alphabet, the natural amino acids, and like human languages, these letters arrange to form secondary structural elements (“words”), which assemble to form domains (“sentences”) that undertake a function (“meaning”). One of the most attractive similarities is that protein sequences, like natural languages, are information-complete: they store structure and function entirely in their amino acid order with extreme efficiency. With the extraordinary advances in the NLP field in understanding and generating language with near-human capabilities, we hypothesized that these methods open a new door to approach protein-related problems from sequence alone, such as protein design.
You can try some of these state-of-the-art method using Colab notebooks from ColabDesing project, again by Sergey Ovchinnikov and colleages (check out his talks here and here).
5 Alphafold3 expanded the scope
Proteins are composed of amino acids, but they also include other components. Holoproteins often contain multiple protein chains, ions, and cofactors. Additionally, proteins’ functions typically involve interactions with various ligands, including DNA or RNA molecules. One limitation of AlphaFold 2 is its ability to predict only the structure of amino acid chains. It generally predicts the orientation of amino acids to some binding pockets accurately, particularly for ions or other common small ligands. Furthermore, it has been demonstrated from the beginning that AF2 can predict protein-protein interactions effectively, which was enhanced with the Alphafold-Multimer version (Evans et al. 2022) and further optimized in methods allowing full interactome predictions (Yu et al. 2023; Molodenskiy et al., n.d.; Rouger et al., n.d.). However, many applications of protein structures in biology, biotechnology, and biomedicine require the interaction of protein chains with other molecules, such as organic ligands, drugs, or DNA/RNA molecules, presenting new challenges in the field of protein prediction.
AlphaFold3 can now predict the structures and interactions of a much wider range of biomolecules, including protein-protein complexes, protein-DNA interactions, protein-RNA interactions, and small molecule-protein interactions. This leap in capability is powered by two key innovations: diffusion models and tokenization. DeepMind proposed that AlphaFold3 has the potential to revolutionize drug discovery, materials science, and our understanding of fundamental biological processes. By accurately modeling the intricate interactions of biomolecules, it can accelerate the development of new therapies, materials, and technologies. For instance, AlphaFold3 could help predict how a person’s specific protein structures and mutations will respond to different drugs, leading to highly effective, personalized treatments. This could revolutionize healthcare, making treatments more precise and reducing the trial-and-error approach currently used. On a global scale, AF3’s predictions could aid in rapidly developing vaccines and treatments for emerging diseases, helping to prevent pandemics and improve global health security. Its impact on neglected diseases could be significant, bringing new hope to millions in underdeveloped regions. This could lead to a more equitable distribution of healthcare advancements, improving quality of life worldwide.
Imagine AlphaFold as the stage of learning to draw a single shape, and AlphaFold2 as mastering the perfection of that shape. AlphaFold3, on the other hand, is like learning to draw that shape and understanding how it fits together with various other shapes to create an intricate and complex picture.
Is AF3 that different?
Unlike AlphaFold2’s direct prediction approach, AlphaFold3 uses a diffusion model, a technique borrowed from image generation. The diffusion process allows the model to consider the overall context of the system, leading to more accurate predictions of complex interactions.
AlphaFold3 (Abramson et al. 2024a) treats biomolecules as “tokens,” similar to how words are represented in natural language processing. This allows the model to apply the same powerful language modeling techniques used in large language models (LLMs). Instead of amino acid sequences, the model works with a broader vocabulary of tokens representing atoms, residues, nucleotides, and small molecules. Each type of atom, residue, or molecule is assigned a unique token. The model learns the statistical relationships between these tokens, capturing the rules of biomolecular interactions. This enables AlphaFold3 to “understand” the language of biomolecules, predicting how they will interact and assemble. Tokenization provides a unified representation for diverse biomolecules, enabling the model to handle complex systems. This, in combination with transformers, allows the model to understand the context of biomolecular interactions, capturing long-range dependencies and intricate relationships.
It is important to note that while AlphaFold3 incorporates the concept of tokenization from language models, it utilizes it within a unique framework for a broader purpose. Tokenization in AlphaFold3 is used to represent all components of a complex, enabling the diffusion model to process these components simultaneously. Unlike protein language models, which primarily focus on learning statistical patterns from large training sets of protein sequences, AlphaFold3 relies on the diffusion model facilitated by tokenization.
Therefore, it is more accurate to describe AlphaFold3 as a hybrid model that integrates principles of language modeling rather than being purely based on protein language models.
Unlike its predecessor, AF3 is a generative model. This means it can give different outputs for the same input and that it can hallucinate, a phenomenon evident with intrinsically disordered regions (IDRs) of proteins.
AF3 can be tested in a Deepmind’s server that allows protein:protein, protein:DNA/RNA interactions, as well as interactions with a small subset of molecules.
Did AF3 move forward the field like AF2?
Short answer: Yes.
There are two main reasons. First, once they published the paper, the community moved to analyze the AF3 workflow and innovations and many labs attempted to reproduce or even improve it. Additionally, like in the case of AF2, Deepmind did not initially release the full code. In this case, they even published the Nature paper without the code. That generated a huge controversy in the social media (again):
There was also some discussion with comments and editorials in Nature and other journals (“AlphaFold3 Why Did Nature Publish It Without Its Code?” 2024; Callaway 2024) and a public letter with more than 100 signatures (Wankowicz et al. 2024). Ultimately, after the Nobel prize concession, they decided to publish an addendum to the paper and release the code on github (Abramson et al. 2024b).
Currently, besides the AF3 released code, there are several AF3-based or AF3 alternatives with similar performance that can be tested by any user. Some examples:
Protenix is a fully opensource AF3 implementation based on PyTorch that allow handling any molecule (Team et al., n.d.)
Chai-1 (Discovery et al., n.d.) is similar to AF3 and it can be used as a Python package for non-commercial use and via a web interface where it can be used for free including for commercial drug discovery purposes. It uses smiles encoding for representation of any ligand molecule
Boltz-1 (Wohlwend et al., n.d.) is also a very recent method that achieves AF3-level accuracy in predicting the 3D structures of biomolecular complexes and it can be tested in Colabfold.
Finally, I recently came into PyMOLFold (Ford et al., n.d.), a novel Pymol plugin that facilitate the use of these methods. It provides instructions for installation (on different Conda environments) and they work on your computer. I tested it using only CPUs and it worked, though quite slowly as compared with the use of online servers or Colabs.
Corollary 2: A Nobel prize to the field
Between 2021 and 2024, I used to conclude my lectures on protein modeling by proposing a wager to my students, predicting that the creators of AF2 and RoseTTAFold would win a Nobel Prize. Indeed, the Nobel Prize in Chemistry for 2024 was awarded to David Baker (1/2) for “computational protein design” and to Demis Hassabis (1/4) along with John Jumper (1/4) for “protein structure prediction”.
As stated by David Baker (Baker and Lin 2025), this Nobel Prize serves as recognition for the field and an encouragement for future advancements, highlighting the significant impact that protein design can have on the world.
6 Review Quizz
AlphaFold and RoseTTAFold are two programs that have been designed to?7 Useful links
Introductory article to Neural Networks at the IBM site: https://www.ibm.com/cloud/learn/neural-networks
ColabFold Tutorial presented presented by Sergey Ovchinnikov and Martin Steineggerat the Boston Protein Design and Modeling Club (6 ago 2021). [video] [slides].
Post about Alphafold2 in the Oxford Protein Informatics Group site: https://www.blopig.com/blog/2021/07/alphafold-2-is-here-whats-behind-the-structure-prediction-miracle/
A very good digest article about the AlphaFold2 paper: https://moalquraishi.wordpress.com/2021/07/25/the-alphafold2-method-paper-a-fount-of-good-ideas/
Post on the AlphaFold revolution meaning in biomedicine at the the UK Institute for Cancer Research website: https://www.icr.ac.uk/blogs/the-drug-discoverer/page-details/reflecting-on-deepmind-s-alphafold-artificial-intelligence-success-what-s-the-real-significance-for-protein-folding-research-and-drug-discovery
A post that explain how AlphaFold and related methods can be used to create new structures: https://theconversation.com/when-researchers-dont-have-the-proteins-they-need-they-can-get-ai-to-hallucinate-new-structures-173209
Selected interesting posts about Alphafold3 modeling process and its potential:
References
Footnotes
See https://www.wired.com/story/without-code-for-deepminds-protein-ai-this-lab-wrote-its-own/↩︎
https://www.bbc.com/news/science-environment-57929095
https://www.forbes.com/sites/robtoews/2021/10/03/alphafold-is-the-most-important-achievement-in-ai-ever/
https://elpais.com/ciencia/2021-07-22/la-forma-de-los-ladrillos-basicos-de-la-vida-abre-una-nueva-era-en-la-ciencia.html↩︎